ML4Print
Automated Forensic Document and Substrate Classification
Despite an increasing level of digitization, important documents can still be found in printed form wherever authenticity is a concern. However, improved scanner and printer technologies are leading to more document forgeries. Counterfeit IDs are integral requirements for e.g. human trafficking, terrorist mobility, cross-border crime or social fraud. Forgeries of birth and marriage certificates lead to obtaining genuine identity documents or government subsidies. Damages of up to €50,000 are easily incurred per forgery. Frontex describes document fraud as one of the biggest challenges in border control in Europe. When detecting document forgery, identifying the source printer and the substrate (paper) used is of great importance. What technique was used to print a document or whether all pages were produced by the same printer and on the same paper is valuable information, even if the primary security features were successfully copied.
This is where the "MLForPrint" project comes in: While manual forensic document examinations can take hours and require many years of experience on the part of the examiner and are therefore used comparatively rarely, the project uses automated processes based on machine learning for this purpose. It is to be shown that software-based and automated examination of printed products and substrates can reduce the examination effort while maintaining comparable accuracy. For this purpose, the project uses so-called Convolutional Neural Networks (CNNs), which have already been used as prototypes to show that an efficient classification of documents with regard to print-related properties such as the printing technique (e.g. offset, dry/wet toner or ink jet) is possible.
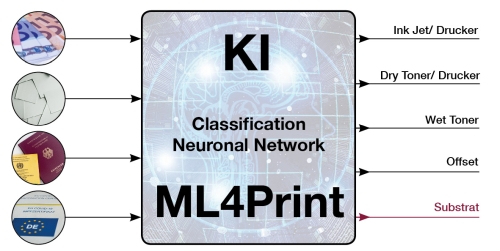
The goals of the "MLforPrint" project are, on the one hand, research into and improvement of the robustness of
the CNN with respect to disturbances that could be specifically exploited by counterfeiters and, on the other hand, the classification
of substrates, for which a software solution is to be demonstrated for the first time that can derive paper types, aging states
and condition predictions from scans.
The challenge of a learned, i.e. data-oriented approach is to be able to react quickly to unknown documents and textures.
For use in digital forensics, it is further important to improve the explainability of the deployed CNN in order to better
understand its decisions and to optimize parameters of the network for deployment purposes.
Key factor of the presented solution is that it reduces effort by up to 80% compared to current methods, and combats damage
and threats caused by using forged or manipulated documents. As a result, the application has a wide field of application
and a large market potential, as there are no comparable systems on the market so far. Typical users are institutions such
as police and registration authorities, BAMF, law enforcement agencies, printers, libraries, archives, art trade as well as
banks and industry.